Vectorize All In One Rag Platform Ai Document Parsing Embeddings Vector Search More
Vectorize All In One Rag Platform Ai Document Parsing Embeddings From lightning fast document parsing to state of the art embeddin vectorize is redefining the way we build rag (retrieval augmented generation) applications!. Vectorize lets you quickly build ai features in your applications powered by your customers' data. integrate with vectorize and you can ingest data from documents, knowledge bases, and saas platforms your customers use.
Multimodal Parsing For Rag Azure Openai Gpt 4o Llamaparse And Azure All in one platform – combines ai document parsing, embeddings, vector search, and retrieval in a single ecosystem. scalability – supports real time and large scale data processing. user friendly – drag and drop ui for seamless pipeline creation. Vectorize helps you build ai apps faster and with less hassle. it automates data extraction, finds the best vectorization strategy using rag evaluation, and lets you quickly deploy real time rag pipelines for your unstructured data. Vectorize is redefining the way we build rag (retrieval augmented generation) applications! from lightning fast document parsing to state of the art embeddings and vector search, vectorize takes care of everything—so you don’t have to. At vectorize, we want to make it fast and easy for our users to create retrieval augmented generation (rag) pipelines to power their ai applications. we’ve tried to make it as intuitive as possible and continue to iterate on our user interface towards this goal.
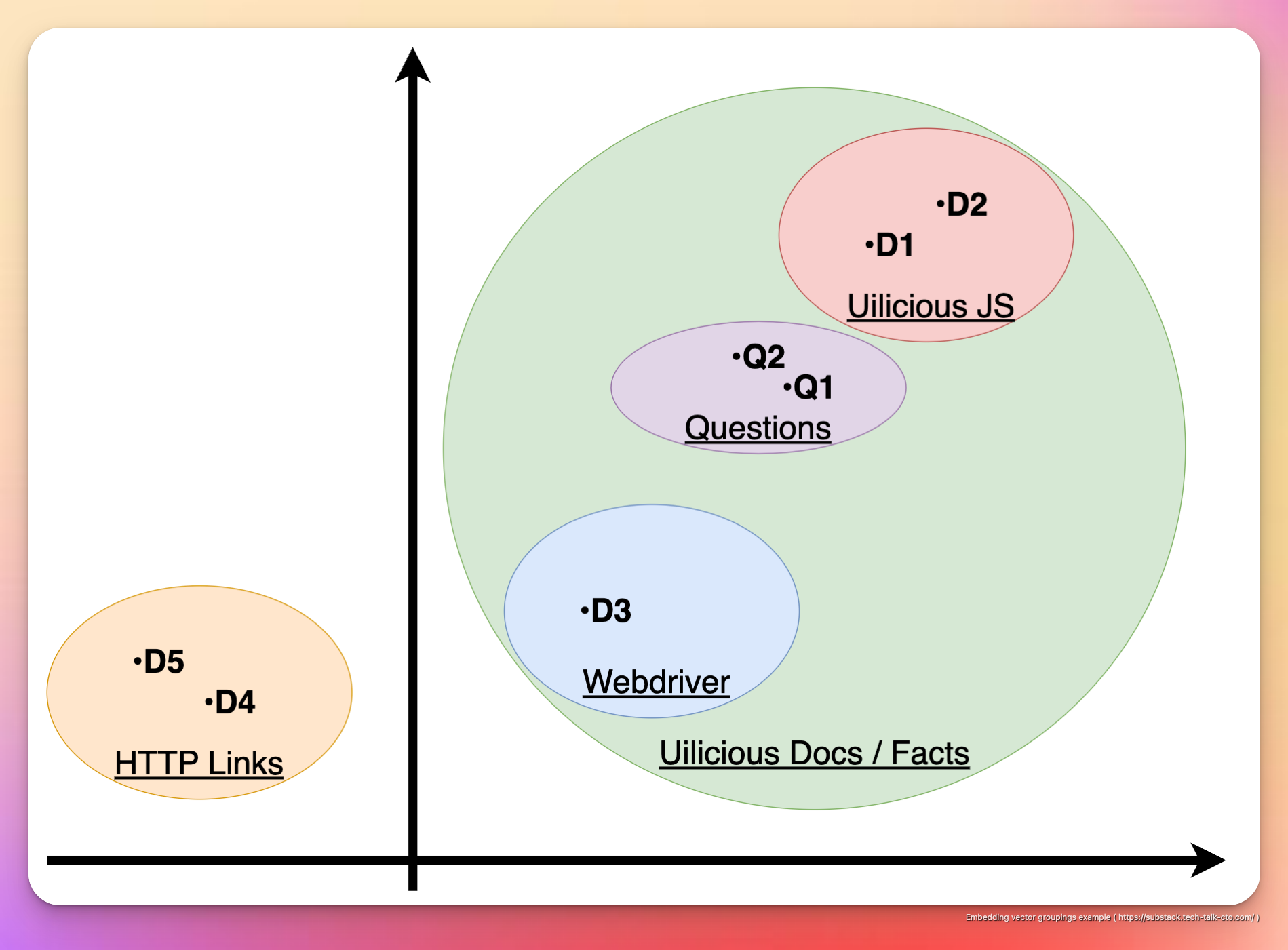
For Example D1 2 3 Are All Documents Related To How To Use Uilicious Vectorize is redefining the way we build rag (retrieval augmented generation) applications! from lightning fast document parsing to state of the art embeddings and vector search, vectorize takes care of everything—so you don’t have to. At vectorize, we want to make it fast and easy for our users to create retrieval augmented generation (rag) pipelines to power their ai applications. we’ve tried to make it as intuitive as possible and continue to iterate on our user interface towards this goal. Rag evaluation starts by providing a sample of the documents you want to use in your rag application and configuring the embedding models and chunking strategies you want to test as part of your evaluation. vectorize then uses your sample documents and this configuration to create and populate a vector index for each of these configurations. Vectorize’s rag pipelines extract and transform unstructured data, load vector search indices into your database, and ensure the indices stay current so your large language model (llm) always has the latest data. by automating your rag pipeline, you can focus on building solid, robust, accurate ai applications. Automatically extract text, images, and tables from pdfs, word docs, powerpoints, and more. get a production quality rag pipeline up and running in minutes. make sure your llm always has the data it needs to give great responses. scale up to millions of documents in your rag pipeline. Through advanced data analysis and streamlined experimentation, vectorize identifies the optimal methods to extract, parse, and vectorize your data, ensuring your llms have access to the most relevant and up to date context.
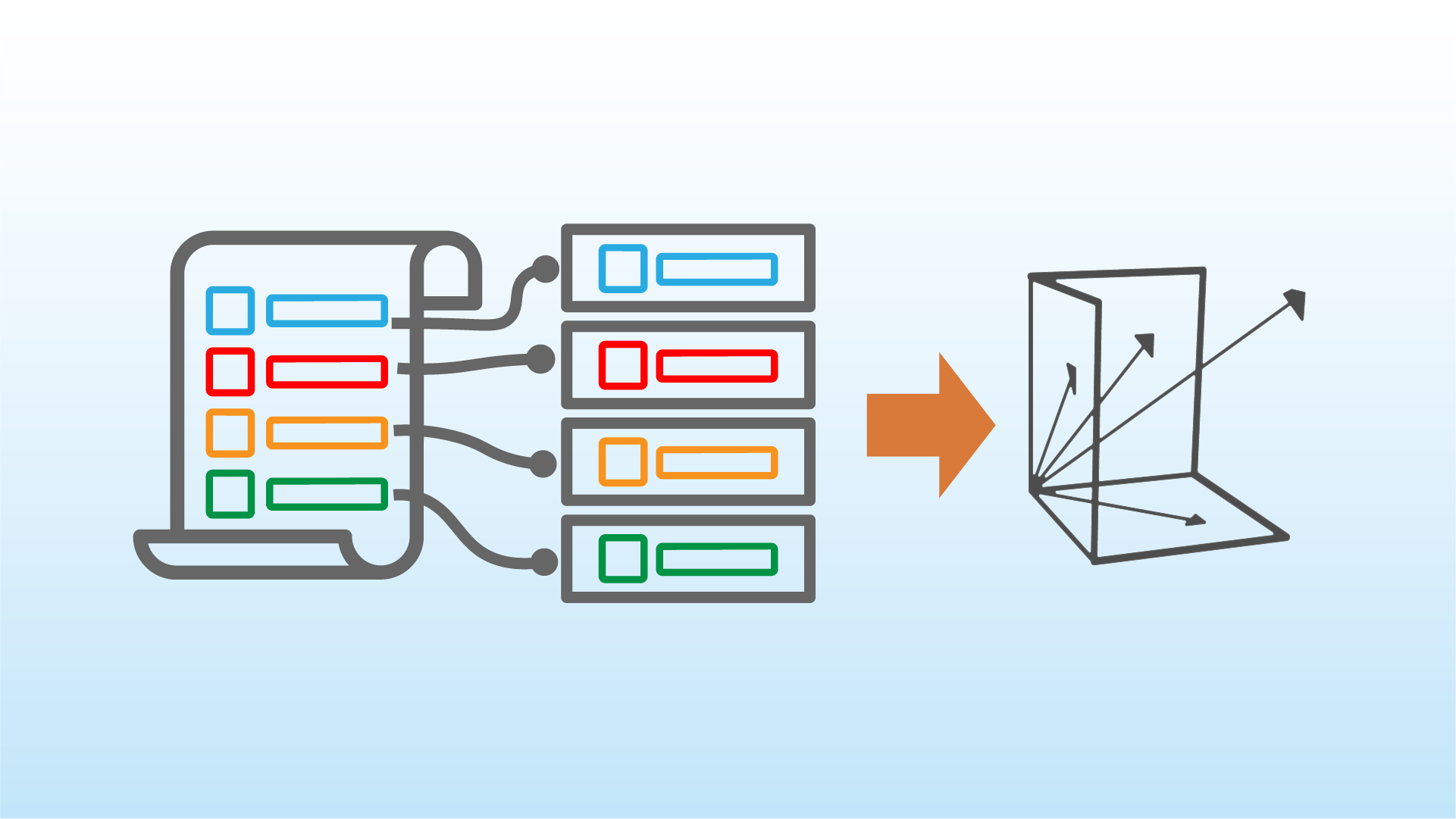
Explore The Role Of Vector Embeddings In Generative Ai Rag evaluation starts by providing a sample of the documents you want to use in your rag application and configuring the embedding models and chunking strategies you want to test as part of your evaluation. vectorize then uses your sample documents and this configuration to create and populate a vector index for each of these configurations. Vectorize’s rag pipelines extract and transform unstructured data, load vector search indices into your database, and ensure the indices stay current so your large language model (llm) always has the latest data. by automating your rag pipeline, you can focus on building solid, robust, accurate ai applications. Automatically extract text, images, and tables from pdfs, word docs, powerpoints, and more. get a production quality rag pipeline up and running in minutes. make sure your llm always has the data it needs to give great responses. scale up to millions of documents in your rag pipeline. Through advanced data analysis and streamlined experimentation, vectorize identifies the optimal methods to extract, parse, and vectorize your data, ensuring your llms have access to the most relevant and up to date context.
Comments are closed.